Abstract
The recent surge in research interest in applying large language models (LLMs) to decision-making tasks has flourished by leveraging the extensive world knowledge embedded in LLMs. While there is a growing demand to tailor LLMs for custom decision-making tasks, finetuning them for specific tasks is resource-intensive and may diminish the model’s generalization capabilities. Moreover, state-of-the-art language models like GPT-4 and Claude are primarily accessible through API calls, with their parametric weights remaining proprietary and unavailable to the public. This scenario emphasizes the growing need for new methodologies that allow learning from agent experiences without requiring parametric updates. To address these problems, we introduce the Experiential Learning (ExpeL) agent. Our agent autonomously gathers experiences and extracts knowledge using natural language from a collection of training tasks. At inference, the agent recalls its extracted insights and past experiences to make informed decisions. Our empirical results highlight the robust learning efficacy of the ExpeL agent, indicating a consistent enhancement in its performance as it accumulates experiences. We further explore the emerging capabilities and transfer learning potential of the ExpeL agent through qualitative observations and additional experiments.
Methodology
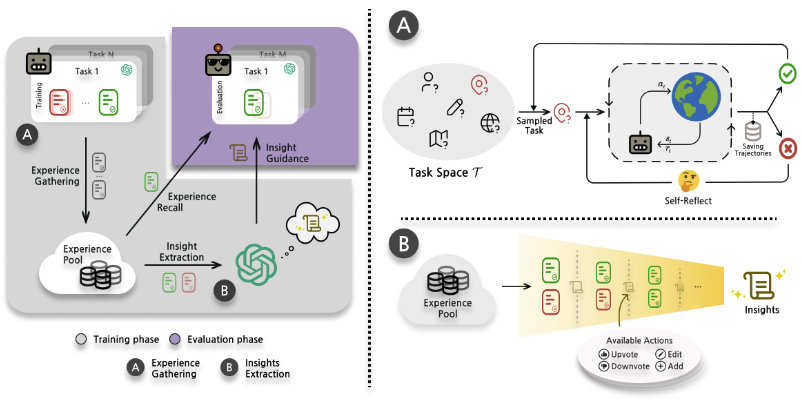
Gathering Experiences
To collect diverse experiences, our method leverages a trial-and-error approach where the agent attempts tasks multiple times. Initially, the agent uses a base planning algorithm with provided fewshot examples and reflects on failed attempts to improve its next try. Successful and failed trajectories are stored in an experience pool, enabling the collection of data that highlights success/failure patterns for future insights.
Learning from Experiences
Our method employs two key learning strategies: (1) recalling successful trajectories from the experience pool based on task similarity, and (2) extracting insights from both successes and failures. Insights are iteratively refined using operations like adding, editing, or voting on their importance, ensuring robust learning from the data.
Task Inference
During evaluation, the agent combines extracted insights and retrieved successful trajectories to augment the task context. This process enhances decision-making by leveraging relevant past experiences and lessons learned, ensuring better performance on new tasks.
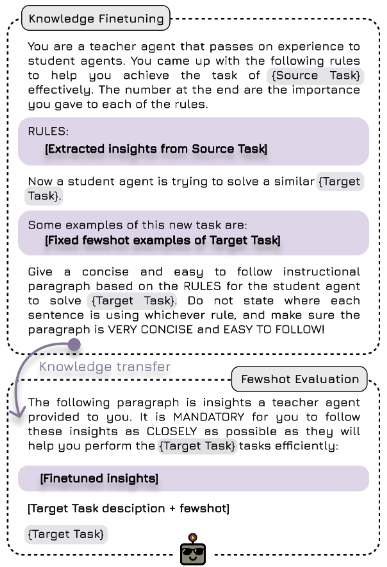
Transfer Learning
Our approach also supports transfer learning by adapting insights from a source task distribution to a target task. Fewshot examples from the target tasks are used to refine the insights, aligning them with the new domain and improving their applicability to unseen tasks.
Results
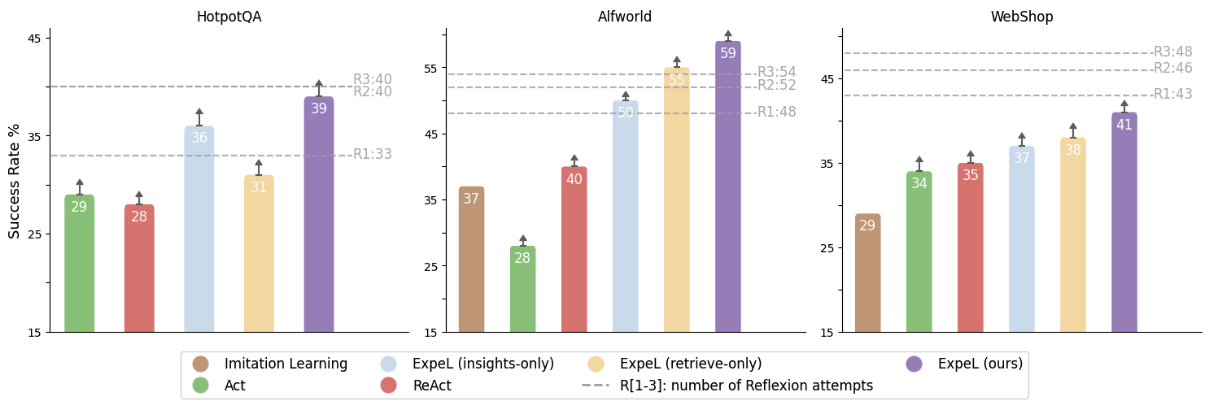
Main Results
Figure above shows that IL-based methods underperform in WebShop and ALFWorld due to limited reasoning capabilities, highlighting the need for knowledge-based models.
Experiential learning improves performance across all tasks. Insights benefit HotpotQA (36%/31%), while trajectory recollection aids ALFWorld (50%/55%). WebShop requires a balance of both, achieving near-equal success rates (37%/38%).
Cross-task Learning
ExpeL matches Reflexion for HotpotQA (40% vs. 39%) and outperforms it in ALFWorld (54% vs. 59%) without repeated attempts. However, Reflexion has higher success rates for WebShop.
Method | FEVER (SR %) |
---|---|
Act | 58 ± 0.0 |
ReAct | 63 ± 0.4 |
ExpeL Transfer w/o Task Demos | 65 ± 1.7 |
ExpeL Transfer | 70 ± 0.7 |
Transfer Learning
ExpeL demonstrates effective transfer learning from HotpotQA to FEVER. Using gpt-4-0613 to adapt insights and finetune with fewshot examples, the agent shows significant performance gains compared to those without in-context examples (see Table above).
Citation
@inproceedings{zhao2024expel,
title={ExpeL: LLM Agents are Experiential Learners},
author={Zhao, Andrew and Huang, Daniel and Xu, Quentin and Lin, Matthieu and Liu, Yong-Jin and Huang, Gao},
booktitle={Proceedings of the AAAI Conference on Artificial Intelligence},
volume={38},
number={17},
pages={19632--19642},
year={2024}
}